Chatbots and virtual assistants continue penetrating our lives. Text prediction and autocorrection tools leave us little to no choice of living without new technologies. This field of AI, also known as natural language processing (NLP), allows machines to generate the language flow, both oral and written. But, before we even start the training process of language models, we need to gather data, create inputs, and more importantly, label them.
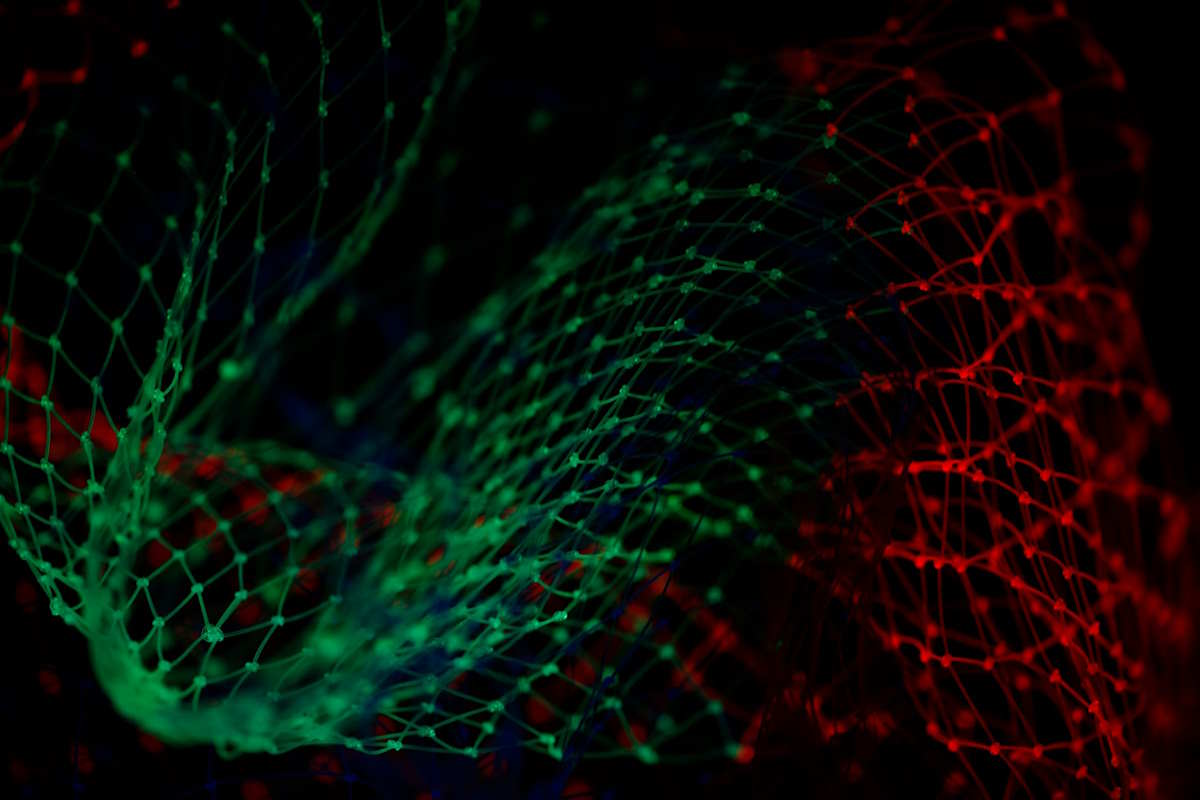
What are the most common linguistic challenges encountered today across industries? How do we overcome them to recognize the needed words and phrases across written texts and audio pieces? How Label Your Data’s solutions move annotation to the next level? Let’s take a close look.
How Do Machines Recognize and Process Language?
Complex algorithms and deep learning not only help machines recognize the text, but also understand, interpret, and finally produce human language. If we take NLP as an example, the language models go through a number of steps before they actually start producing the language. The most common ones include:
- Text pre-analysis. At the stage of pre-analysis, or preprocessing, there is the split of text into the smaller pieces, which is called tokenization. For better understanding, the text is divided into phrases and words. At this stage, we normalize the text, making it simpler, removing all special characters or punctuation. We then eliminate unnecessary words that don’t change the meaning, and reduce the rest to its root simple form.
- Syntactic and semantic analysis. At this step, the algorithms analyze the grammar structure and the role of the word in the sentence. They also try to understand the meaning in the next, identifying any ambiguous words, categorizing them, and associating the relationship between the words in the text.
- Sentiment analysis. The sentiment analysis helps to identify the intention and the sentiment of the speaker or writer, which can be positive, negative, or neutral.
- Training. With the help of deep learning techniques, we can train language models to perform various tasks. After the pre-analysis and processing of the text, the model “learns” to perform such tasks as classification, prediction, and text generation.
- Language generation. After all stages of training, the NLP can finally produce the language, creating coherent and meaningful sentences based on the initial input.
You’ll ask where in all this journey stays data annotation? It’s related to the very first input you’ll provide to the model and ensures the accuracy of AI-generated outputs. Let’s see the primary functions in data annotation and how the team of experts at Label Your Data, the NLP services provider, helps to leverage the understanding of data.
Role of Data Annotation in ML’s Language Understanding
Before you start the process of the ML model training, your data for the input should be collected, categorized, and well annotated. Data annotation plays one of the most important roles in enabling ML models to understand and process data. Here are its main functions:
- Preparation for the training. Data annotation involves labeling raw data (either text or speech) with relevant tags or labels. They usually identify specific features or attributes relevant to the learning task. These annotations help ML models learn the patterns needed to generate human language.
- Improving accuracy. The more accurately and comprehensive the annotated data, the better the model translates the human language. Accurate data annotations enable models to discern subtle nuances in language. This improves language translation, question answering tasks, and sentiment analysis.
- Enhancing context. Annotations can highlight idiomatic expressions, sarcasm, or cultural references. They remain crucial for models to grasp the intended meaning in complex language use cases.
- Reducing biases. Careful and diverse data annotation can help in identifying and mitigating biases in NLP. The more diverse and detailed data is included in the input, the more accurate and correct will be the output.
- Facilitating improvement. Annotated data supports the initial training of NLP models and also contributes to their improvement. Continuous improvement makes models adaptable to new languages, dialects, or emerging uses of language.
Challenges of Understanding Language Datasets Across Industries
Understanding language datasets across various industries involves unique challenges that arise due to the inherent complexity of human language. Add here the jargon and the context-dependent nature of language understanding, and the machine will be lost. For the training, NLP uses raw datasets that include huge amounts of information. The biggest challenge is its low quality and ambiguity, leading to incorrect outputs.
Put the data annotation aside, and the generation of human language will become impossible for ML. Take an example from the healthcare sector. It’s full of medical records and literature filled with complex terminology and abbreviations. Another example is the legal industry. Legal documents usually contain formal language and complex sentence structures. Data annotation helps differentiate all these nuances and put precision tags for further machine learning training.
Solutions Offered by Label Your Data
The team of experts at Label Your Data offers various data annotation services. They work with various industries and with data of various difficulty levels. The common tasks range from semantic segmentation to transcription to image categorization, to name just a few. With the usage of labeling tools, the team works with multiple languages. The whole process of annotation starts with collecting data and finishes with QA.
The annotation process is literally converting the unlabeled data with tags or labels. They will meet the requirements for the further usage of data by the ML algorithms. Human annotation helps to get higher precision and better accuracy. Such a diligent approach allows annotating even the most complicated and cumbersome data.
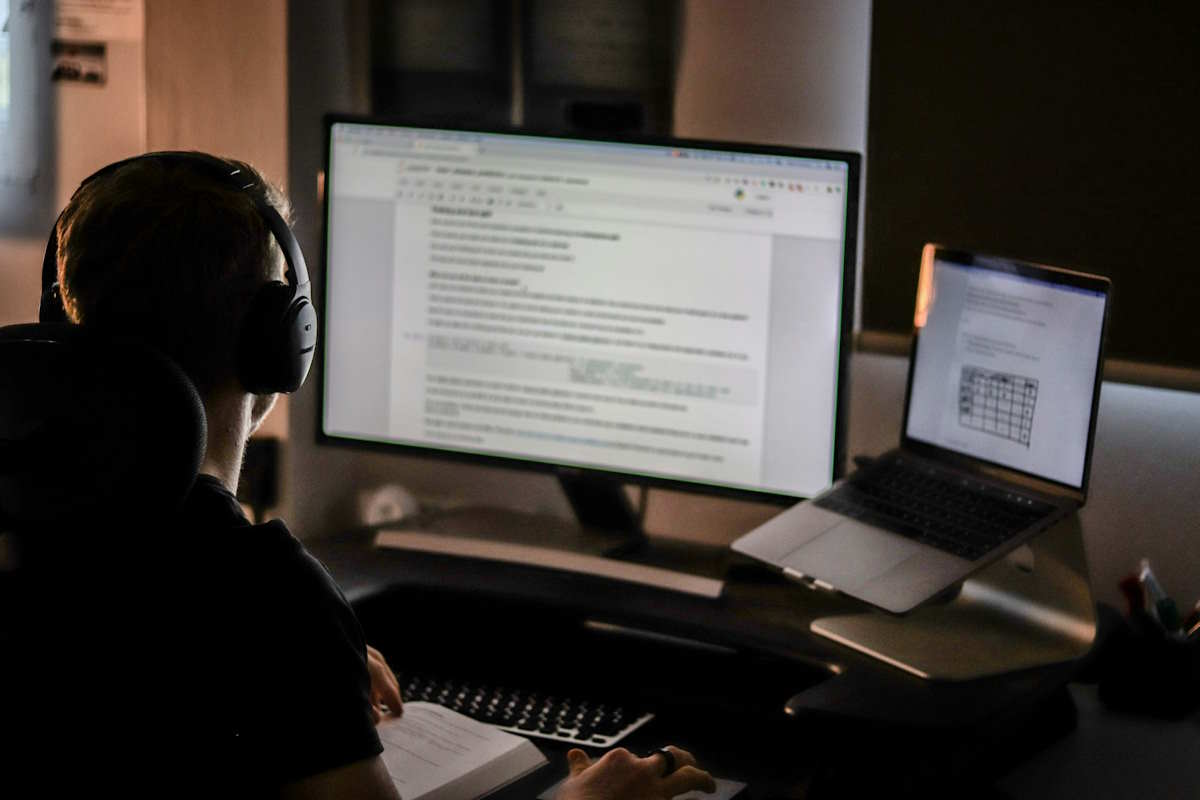
Core Insights
The magic of every chatbot lies in its extensive and complex training process. Before the ML model can mimic human language and engage in conversation, it undergoes several training phases.
Today’s primary challenge is dealing with unlabeled, ambiguous, or poor-quality data. This underscores the importance of data annotation prior to implementing ML algorithms. Human annotation services provide high-quality annotations that take into account the industry, language, and specific requirements of the ML task. Correctly annotated data is now a key factor in the success of ML algorithms.